New applications of machine learning have enabled astronomers to classify 27 million galaxies and pick out a dozen rare quadruply lensed quasars.
Two new studies use machine learning — a type of artificial intelligence — to achieve astronomical feats difficult, if not impossible, for humans to do on their own.
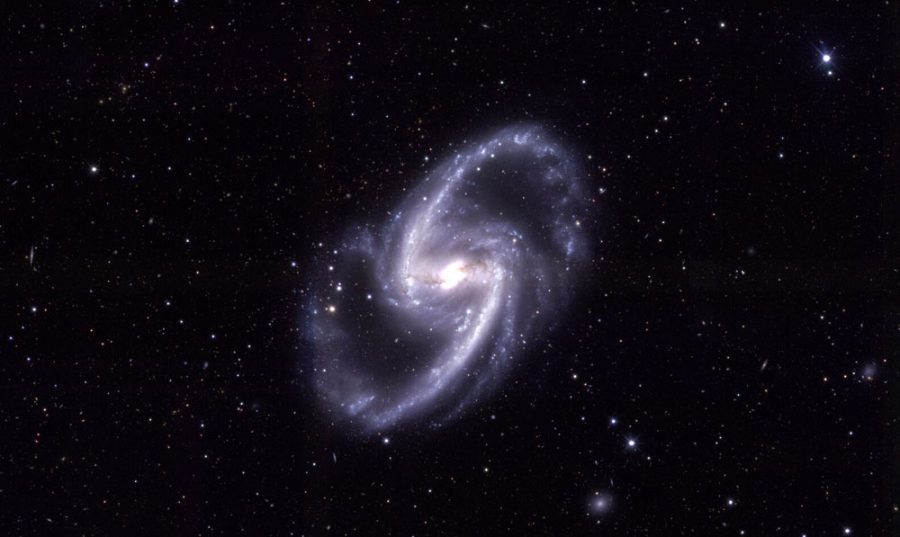
DECam / DES Collaboration
When faced with millions of galaxies observed as part of the Dark Energy Survey (DES) at the Cerro Tololo Inter-American Observatory in Chile, Jesús Vega-Ferrero (University of Pennsylvania) and colleagues turned to convolutional neural networks, the same kind of computer model used in facial recognition. Like a set of computerized sorting hats, these networks decided each galaxy’s place in two categories: shape (spiral or elliptical) and orientation (face-on or edge-on).
The group had previously worked with bright galaxies from the Sloan Digital Sky Survey (SDSS), but the DES goes deeper, finding galaxies 1,000 times fainter. So the researchers trained their neural networks to go further, too. First, they taught the computer models using a real set of galaxies that were in both the SDSS and DES surveys, but these galaxies were still bright. To teach the networks to classify even fainter galaxies, the team “aged” SDSS galaxies so that they’d appear farther away. Once trained on these real and simulated data, the neural nets were set loose on all the DES galaxies.
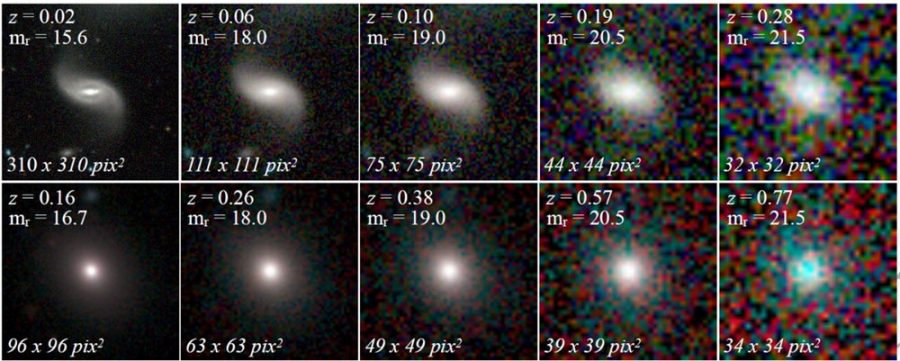
Jesus Vega-Ferrero and Helena Dominguez-Sanchez
The result, 27 million galaxy classifications, is beyond even crowdsourced human ability. In the Galaxy Zoo citizen science project, volunteers took about two years to classify a million galaxies (with multiple people classifying each galaxy). An impressive number, and one that only grew in the following decade — but still a drop in the ocean of Big Data about to engulf astronomy from multiple facilities on the ground and in space.
Machine-learning isn’t foolproof, though. Testing against a subset of the galaxies used for training the neural networks showed that they were 97% accurate. But the computer models also rank their own work, and their confidence level depends on multiple things, including the type of galaxy being qualified. Overall, the networks are less sure about roughly 15% of the spiral/elliptical classifications and 27% of the face-on/edge-on classifications.
Diamonds in the Rough
The European Space Agency’s Gaia mission is another Big Data facility. While mapping a billion stars in the Milky Way, the satellite has also picked up millions of quasars, galaxies that host a gas-slurping supermassive black hole in their brilliant centers. But not every quasar is equally interesting. On rare occasions, when galaxies align just right, a foreground galaxy can gravitationally lens a distant quasar, producing four images, sometimes dubbed an Einstein’s Cross.
These “diamonds in the rough” are not only beautiful cosmic coincidences; they are also tremendously useful in obtaining an independent measure of the universe’s current expansion rate, a value that astronomers are currently debating.
It has taken four decades to find 56 Einstein Crosses. Now a project that subjects Gaia data to multiple machine-learning methods has picked out another dozen in one fell swoop. The team, led by Daniel Stern (JPL-Caltech), used multiple ground-based observatories to confirm the finds.
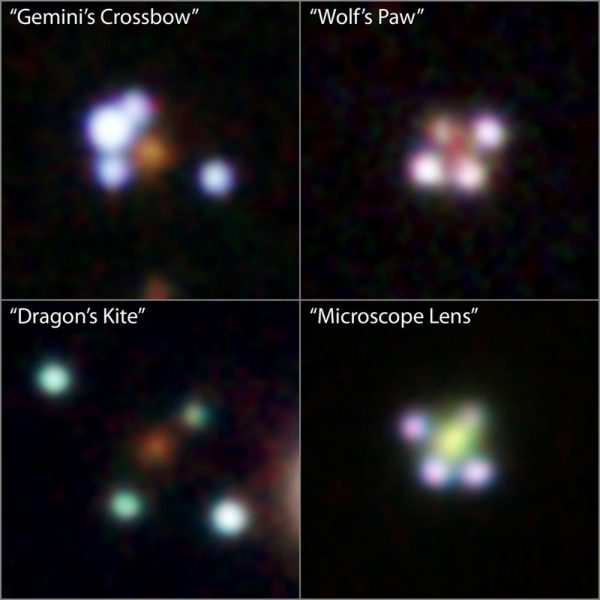
The GraL Collaboration
The ever-so-slightly asymmetric lenses have a personality all their own, and the researchers took full advantage of the discoveries to offer nicknames based on a quad’s appearance and its position in the sky. For example, the closely spaced “Wolf’s Paw” is found in the constellation Lupus, the Wolf, and looks just like its namesake. Another, “Dragon’s Kite,” is in Ophiuchus, the Serpent Bearer.
In addition to describing the confirmed finds, the team also points out asterisms, the ones that looked like quadruple lenses but turned out to be something else. Most often, the algorithms were fooled when foreground stars in our own galaxy masqueraded as one or more distant quasar images. This “ground truth” offers the team a chance to revise the machine-learning methods before applying them to the newest Gaia data release.
“Machine learning was key to our study, but it is not meant to replace human decisions,” explains team member Alberto Krone-Martins (University of California, Irvine). “We continuously train and update the models in an ongoing learning loop, such that humans and the human expertise are an essential part of the loop. When we talk about 'AI' in reference to machine-learning tools like these, it stands for Augmented Intelligence not Artificial Intelligence.”
References
J. Vega-Ferrero et al. “Pushing automated morphological classifications to their limits with the Dark Energy Survey.” Monthly Notices of the Royal Astronomical Society, 2 March 2021. (preprint available here)
D. Stern et al. “Gaia GraL: Gaia DR2 Gravitational Lens Systems. VI. Spectroscopic Confirmation and Modeling of Quadruply-Imaged Lensed Quasars.” To appear in the Astrophysical Journal. (preprint available here)
Comments
Joe Adlhoch
April 16, 2021 at 4:50 pm
Monica, I'm curious why there are 4 images of these gravitationally lensed quasars and not 3 or 5 or a ring around the foreground galaxy. And what can we tell about the type and orientation of the foreground galaxy based on the distribution of the lensed quasar components?
Also, I'm curious what the visual magnitude of these examples are. I have seen 2 of the components of the quad gravitationally lensed quasar named Andromeda's Parachute with a large dob. But that one is "fairly bright" as lensed quasars go. Are any of these accessible to amateurs?
You must be logged in to post a comment.
Monica YoungPost Author
April 21, 2021 at 10:31 am
Hi Joe, good questions! The four images occur because of the precise (albeit happenstance) position of the quasar behind the foreground galaxy. Sometimes you can get three images, if one of the four images is too faint to see. I don't believe five images are possible — if the quasar is a little out of line with the foreground galaxy, it instead smears out into a ring.
The G-band (Gaia's band, which very roughly corresponds to V band) magnitudes of the quadruply lensed quasars referenced here are 18th to 20th, so these sources are unfortunately out of reach for backyard telescopes.
You must be logged in to post a comment.
You must be logged in to post a comment.